Power BI Blog: Key Influencers
14 February 2019
This week in Power BI, we’re going to look at a brand new feature that’s just been released, called “Key Influencers”.
This is a new visual that allows you to quickly evaluate what parameters in your dataset result in significant swings in other parameters. It’s a simple way of segmenting your data in a way that makes it easy to see how certain subsets of your data are highly correlated with key parameters that you might be looking at.
Here’s a simple way of explaining how it works: a bank might have 10 million customers. It only has 1 million customers who took out a new loan in the last 12 months. On average therefore, only 10% of customers take out a new loan over a 12 month period. However, if you only look at customers that have more than $50,000 in their savings accounts, then that likelihood might jump from 10% to 25% of customers.
With this information, you might be able to better target any marketing to this customer subset (i.e. only people with balances of more than $50,000), or you might work to help get other customers to save up and achieve higher balances, so that you have more customers in that subset.
With our sample Power BI dataset, we might want to look at characteristics that influence large sales – those over $3,000 per transaction. We can create a category that defines the behaviour that we’re looking for and look through a list of different fields to see what are the influencing factors.
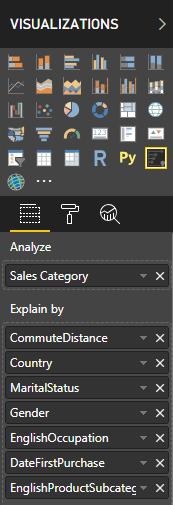
In this instance, we’ve defined a Sales Category field that looks at sales over $3,000 in value. Then, we’ve asked the Key Influencers visualisation to explain what the likelihood of achieving that is, based on a range of different items: commute distance, country, marital status, gender, occupation, when a customer’s first purchase was, and the type of product that was sold.
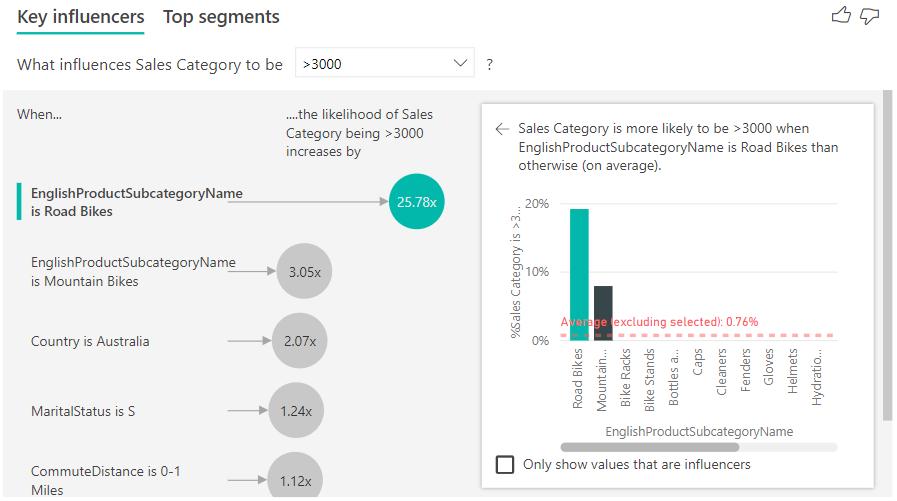
Clearly, Road Bikes and Mountain Bikes top the list, with nearly 20% of road bikes costing over $3,000 and around 8% of mountain bikes meeting the criteria. However, we can also see some other interesting things, such as the fact that single people (those with a marital status of S) are 24% more likely to buy an expensive bike than married people.
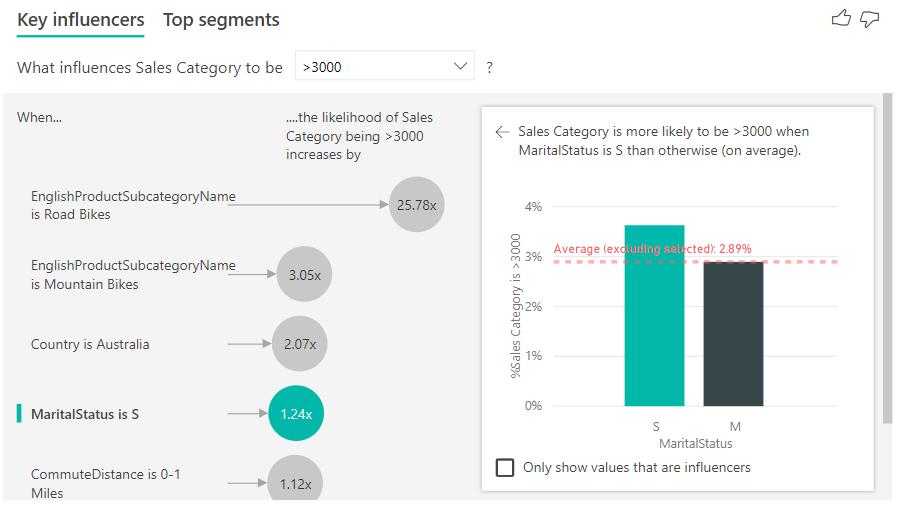
This visualisation is still in preview mode, however, it is an exciting advancement in what Power BI can do in the analytics space. We’re going to spend the rest of this month playing around with this feature and exploring the insights that you can achieve from this new tool. See you next week!